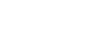
Add the Title Text Here
read about our project
OUR MISSION
Learning around Algorithmic Technologies in Medicine
The LIAISON project is a collaboration of organizations focussed on moving towards the effective deployment of novel algorithmic technologies in healthcare. By facilitating a learning community of diverse stakeholders in medical AI, LIAISON promises a collective learning journey across and beyond occupational boundaries.






OUR COSORTIUM



The effective development and deployment of medical AI still forms a problem for many diverse stakeholders.
The LIAISON learning community forms a space where communities of Medical Practitioners, Technology Developers, Governance & Policy Actors, and Patients come together to shift the state of medical AI from a technology problem into a learning challenge.

MEDICAL
PATIENTS
POLICY
TECHNOLOGY
OUR LEARNING COMMUNITY
OUR LEARNING ENGINE
%20(0)_4x.png)
how it works
At the core of our learning community, we have what we call a learning engine, a 3-step process that drives the generation of systemic outcomes and impacts through community learning.

Curious to learn more about our project? Or interested in joining our learning community?
Contact us using the form below
or send an email to liaison@vu.nl
CONTACT US



STEP ∞ : UNLIMITED ITERATION
Given the cyclical design of the collaborative learning process, the insights from step 3 can feed into new scenarios for experimentation, which facilitate new experiments, which facilitate new discussion...

STEP 3: COLLECTIVE DISCUSSION
In this last step, the results of the experiments are analyzed and discussed in a multi-stakeholder engagement. In this step, the insights from experiential learning are reinforced with a process of reflective, critical learning, which offers the basis of a new cycle.



STEP 2: COLLABORATIVE EXPERIMENTATION
In this step, the determined scenarios are emulated in experiments simulating real-life conditions, to facilitate experiential learning processes when engaging with medical AI without any ethical or safety concerns.



STEP 1: CO-DESIGNING SCENARIOS
In this step, specific scenarios of human-AI interaction are designed together with the 4 communities of stakeholders. These scenarios should be socio-economically critical, medically relevant, technologically feasible, and ethically and legally compliant.


